Introduction
In the realm of finance, forex markets reign supreme, facilitating colossal currency exchanges worth trillions of dollars daily. Harnessing the immense data churned by these markets has become a holy grail for traders, enabling them to decipher patterns, forecast trends, and make informed decisions. Enter machine learning, a game-changer that elevates forex analysis to unprecedented heights.
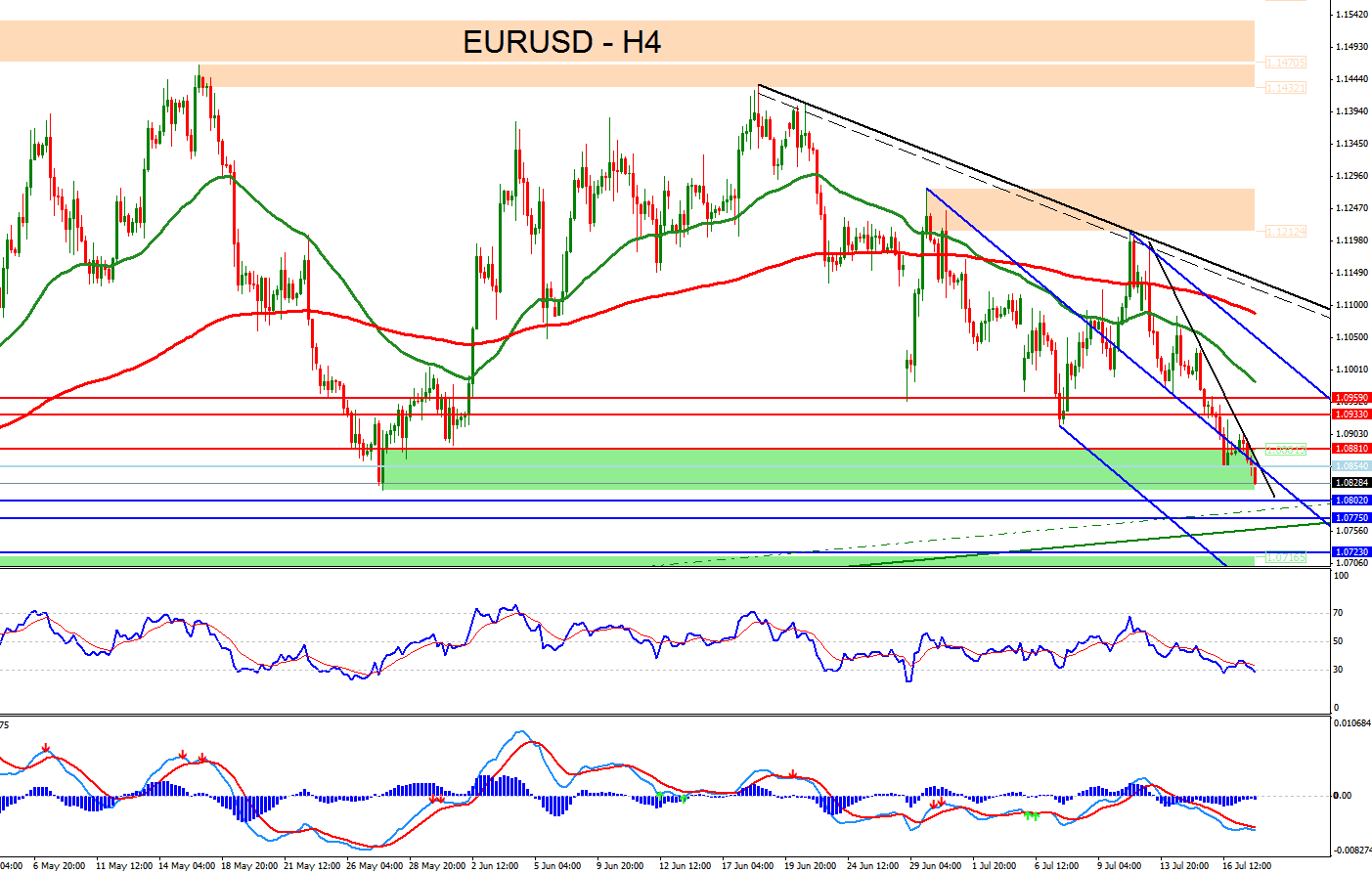
Image: forexsignalsmarket.blogspot.com
Machine learning models, empowered by sophisticated algorithms, can unearth hidden correlations, uncover anomalies, and learn from vast datasets. Forex data, with its constant price fluctuations, is a goldmine for these models, transforming them into powerful predictive tools. In this article, we’ll delve into the world of forex datasets for machine learning, exploring their applications, challenges, and the remarkable possibilities they unlock for traders.
Section 1: A Goldmine of Forex Data
Forex data encompasses a myriad of variables, including currency pairs, historical prices, trading volume, and economic indicators. This data can be gathered from various sources, including brokers, data aggregators, central banks, and online platforms. Historical data can span years, providing invaluable insights into market trends and seasonality. Live data feeds, on the other hand, deliver real-time updates, enabling traders to monitor market movements and execute trades promptly.
Section 2: Unleashing the Power of Machine Learning
Machine learning algorithms, fed with vast forex datasets, can learn intricate relationships within the data and make predictions. These algorithms can identify patterns, forecast price movements, optimize trading strategies, and manage risk more effectively. While numerous machine learning algorithms exist, some commonly used in forex include linear regression, decision trees, random forests, and neural networks. The choice of algorithm depends on the specific trading goals and the characteristics of the dataset.
Section 3: Building Robust Forex Models
Constructing robust machine learning models requires a systematic approach. Data preprocessing is crucial, involving cleaning, normalizing, and transforming the raw data to enhance the model’s performance. Feature selection plays a critical role, identifying the most influential variables from the dataset to train the model. Once trained, the model should be rigorously evaluated using metrics like accuracy, precision, and recall to assess its predictive capabilities.
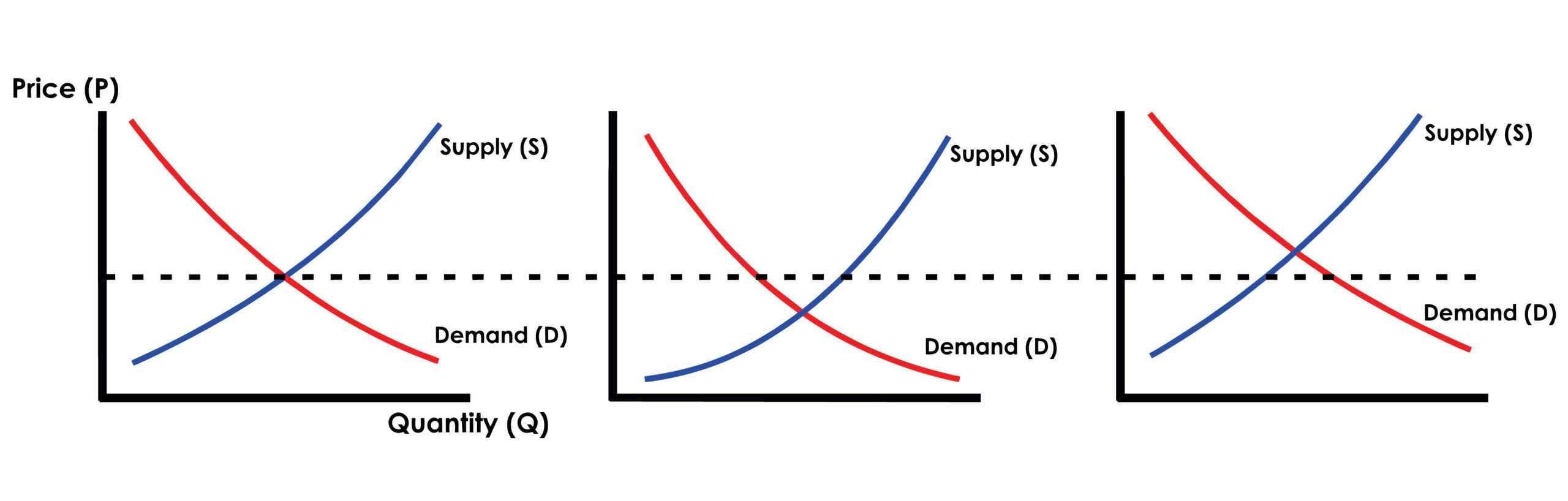
Image: mytradingskills.com
Section 4: Real-World Applications
Forex datasets for machine learning empower traders with a wealth of practical applications. They can:
- Forecast currency exchange rates and identify trading opportunities
- Develop automated trading systems that execute trades based on pre-defined rules
- Optimize trading strategies to maximize profit potential and minimize risk
- Identify market anomalies and price manipulation, helping traders avoid potential pitfalls
- Conduct risk analysis and implement risk management strategies to protect capital
Section 5: Challenges and Limitations
While forex datasets offer immense potential, they also present certain challenges. Data quality can vary significantly, necessitating careful selection of reliable data sources. Overfitting, a situation where a model performs exceptionally well on training data but poorly on unseen data, can be a concern. Additionally, machine learning models rely on historical data and may not capture sudden market shifts or unforeseen events.
Forex Dataset For Machine Learning
Section 6: Conclusion
Forex datasets, combined with the power of machine learning, provide traders with an arsenal of tools to enhance their trading decisions. By harnessing the wealth of data available, traders can gain a deeper understanding of market dynamics, identify trading opportunities, and optimize their strategies. As machine learning continues to evolve, its integration with forex data promises even more remarkable advancements, revolutionizing the way traders navigate the ever-changing forex markets.